Machine Learning Prediction of Postoperative Emergency Department Hospital Readmission
Abstract
Background
Although prediction of hospital readmissions has been studied in medical patients, it has received relatively little attention in surgical patient populations. Published predictors require information only available at the moment of discharge. The authors hypothesized that machine learning approaches can be leveraged to accurately predict readmissions in postoperative patients from the emergency department. Further, the authors hypothesize that these approaches can accurately predict the risk of readmission much sooner than hospital discharge.
Methods
Using a cohort of surgical patients at a tertiary care academic medical center, surgical, demographic, lab, medication, care team, and current procedural terminology data were extracted from the electronic health record. The primary outcome was whether there existed a future hospital readmission originating from the emergency department within 30 days of surgery. Secondarily, the time interval from surgery to the prediction was analyzed at 0, 12, 24, 36, 48, and 60 h. Different machine learning models for predicting the primary outcome were evaluated with respect to the area under the receiver-operator characteristic curve metric using different permutations of the available features.
Results
Surgical hospital admissions (N = 34,532) from April 2013 to December 2016 were included in the analysis. Surgical and demographic features led to moderate discrimination for prediction after discharge (area under the curve: 0.74 to 0.76), whereas medication, consulting team, and current procedural terminology features did not improve the discrimination. Lab features improved discrimination, with gradient-boosted trees attaining the best performance (area under the curve: 0.866, SD 0.006). This performance was sustained during temporal validation with 2017 to 2018 data (area under the curve: 0.85 to 0.88). Lastly, the discrimination of the predictions calculated 36 h after surgery (area under the curve: 0.88 to 0.89) nearly matched those from time of discharge.
Conclusions
A machine learning approach to predicting postoperative readmission can produce hospital-specific models for accurately predicting 30-day readmissions via the emergency department. Moreover, these predictions can be confidently calculated at 36 h after surgery without consideration of discharge-level data.
Editor’s Perspective
What We Already Know about This Topic
- Unplanned hospital readmissions are a focus of quality improvement, national benchmarking, and payment incentives in the United States
- The accuracy of commonly used peer-reviewed readmission prediction algorithms at specific hospitals may be limited by hospital-specific factors
- The potential value of novel machine learning techniques capable of incorporating hundreds of patient, process, and hospital attributes is unclear
What This Article Tells Us That Is New
- Hospital-specific 30-day surgical readmission models using machine learning techniques provide clinically usable predictions when applied to future patients
- A parsimonious approach limiting which data elements are considered performs as well as more comprehensive models
Topics:
emergency service, hospital, machine learning, patient readmission, surgical procedures, operative, laboratory, trees (plant)
Unplanned hospital readmissions have been a pressing concern with respect to patient burden and high cost.1 These factors are compounded when a patient is readmitted via the emergency department where they may consume valuable resources. This is in contrast to postoperative readmissions that occur via direct admission after clinic visits that are often planned or facilitated by a surgeon. Although it is not always clear whether a given readmission is preventable, it is certain that emergency department–based 30-day readmissions are sentinel events, poor markers of quality, and are typically attributable to conditions present at discharge.2,3
Models created to detect patients at risk for unplanned readmission have been developed, but many of them have a narrow focus on single disease states and cannot be applied to the postoperative population as a whole.4–6 Furthermore, most of these models were exclusively created for nonsurgical patients, making them difficult to validate in different cohorts,7 and many of them require substantial data from the moment of hospital discharge.8,9 Waiting until hospital discharge inhibits providers from better optimizing factors associated with readmission in parallel with the routine postoperative care.4,10–12 This delay can also lead to ineffective transitional care coordination or possible prolongation of a hospital stay. Moreover, given that timeliness is one of the five rights of clinical decision support, waiting until the moment of discharge dramatically decrease the clinical efficacy of any model.13
A potential path to developing patient-risk models is machine learning with its ability to process extremely large numbers of input features and produce accurate predictive models.14,15 More specifically, tree-based machine learning methods are able to model nonlinear relationships and interactions, typically outperforming standard logistic regression.16 Lastly, machine learning models which are calibrated using an institution’s individual data demonstrate higher accuracy than models engineered for generalized patient cohorts that ordinarily overlook unforeseen institution-specific nuances.17
In this study, we describe the creation and comprehensive validation of a machine learning–based methodology for predicting a patient’s risk for 30-day readmission via the emergency department in the postoperative period. Our primary hypothesis is that machine learning methods are capable of producing hospital-specific readmission prediction models with excellent discrimination. Our secondary hypothesis is that machine learning can produce models that accurately predict postoperative readmission without dependence on data from the time of discharge.
Ready to transform your data
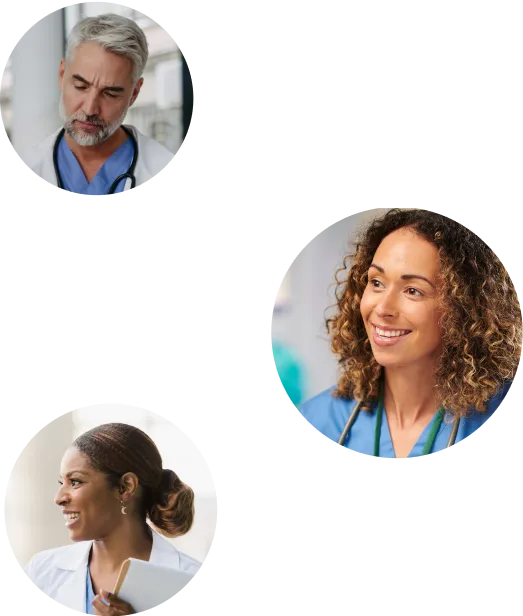
.webp)