Integration of feature vectors from raw laboratory, medication and procedure names improves the precision and recall of models to predict postoperative mortality and acute kidney injury
Abstract
Manuscripts that have successfully used machine learning (ML) to predict a variety of perioperative outcomes often use only a limited number of features selected by a clinician. We hypothesized that techniques leveraging a broad set of features for patient laboratory results, medications, and the surgical procedure name would improve performance as compared to a more limited set of features chosen by clinicians. Feature vectors for laboratory results included 702 features total derived from 39 laboratory tests, medications consisted of a binary flag for 126 commonly used medications, procedure name used the Word2Vec package for create a vector of length 100. Nine models were trained: baseline features, one for each of the three types of data Baseline + Each data type, (all features, and then all features with feature reduction algorithm. Across both outcomes the models that contained all features (model 8) (Mortality ROC-AUC 94.32 ± 1.01, PR-AUC 36.80 ± 5.10 AKI ROC-AUC 92.45 ± 0.64, PR-AUC 76.22 ± 1.95) was superior to models with only subsets of features. Featurization techniques leveraging a broad away of clinical data can improve performance of perioperative prediction models.
© 2022. The Author(s).
Conflict of interest statement
The authors declare no competing interests.
Ready to transform your data
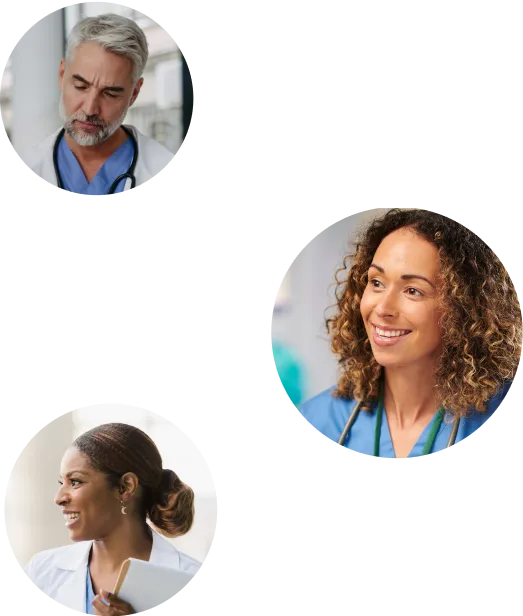
.webp)